Are We Ready to Sell AI Cars?
In October, the automotive industry saw a significant surge of excitement as both Xpeng and Tesla unveiled their respective AI vehicles. The competitive landscape is heating up incredibly fast, sparking interest and speculation about the future of intelligent mobility. To understand the implications, we have to roll back time, four years to be specific.
In 2020, two prominent figures in the automotive sphere, He Xiaopeng of Xpeng and Elon Musk of Tesla, found themselves embroiled in a public dispute. Xpeng declared its intentions to implement LiDAR technology in its upcoming models to enhance autonomous driving capabilities. This technology serves as an environmental perception system for AI-enabled vehicles, aiming to elevate the safety and reliability of autonomous navigation.
Musk, never one to shy away from a spoken jab, retorted from across the ocean, criticizing Xpeng for allegedly plagiarizing outdated codes from Tesla and Apple. He confidently asserted that while Xpeng might have access to an older version of Tesla's software, they lacked Tesla's state-of-the-art neural networks, indicating a technological disparity that could spell disaster for the Chinese company.
In response, He Xiaopeng took to social media to vehemently counter Musk’s claims, claiming his company’s innovation stemmed from its own research and development rather than imitation. Many observers at the time dismissed their clash as a mere spectacle, not foreseeing that this rivalry would mature into a real competition.
Advertisement
Fast forward to October of this year, Xpeng made headlines once again when it rebranded itself as "Xpeng AI Automotive Company." On October 10, the company released its new model, the "Xpeng P7+," claiming the title of "the world's first AI car," with a pre-sale price starting at approximately 209,800 RMB and an anticipated launch date in early November.
On the same day, in Los Angeles, a place synonymous with tech innovation, Musk unveiled Tesla's highly anticipated autonomous taxi, the Cybercab. This simultaneous launch from both industry leaders indicates a trend that seems unstoppable—the integration of AI into the fabric of modern automobiles is rapidly becoming the norm.
In April of this year, Musk made a short trip to China, heightening speculation about the introduction of Tesla’s Full Self-Driving (FSD) technology within the nation. During a subsequent earnings call, he hinted that the FSD was likely to gain approval in both China and Europe by year's end. This countdown adds immense pressure on domestic electric vehicle manufacturers, who find themselves in direct competition with an established player like Tesla.
Traditionally, Tesla has occupied a niche within the realm of intelligent driving—often described as the “minority” among competitors. In August of last year, the company celebrated the release of its FSD Beta V12, which it touted as the world’s first end-to-end AI autonomous driving system. So, what does “end-to-end” really mean? In layman's terms, it refers to a groundbreaking approach where artificial intelligence models replace traditional modules for perception, planning, and control. This means that the vehicle operates not via codes written by engineers but through algorithms that simulate human thought processes.
This shift generated considerable buzz within the industry, prompting various automakers and autonomous driving companies to initiate their own researched developments of end-to-end technologies. The domestic landscape experienced a notable shift as established companies began paving their own paths toward intelligent driving approaches.
By the second half of 2023, NIO established a dedicated team solely for the development of end-to-end models. Li Auto’s Vice President of Intelligent Driving, Lang Xianpeng, noted that they had begun research into end-to-end technology development long before Tesla's FSD V12 announcement, suggesting confidence in their path ahead. As we inch closer to 2024, multiple car manufacturers have commenced integrating such technologies into their vehicles.
In April, Huawei unveiled its Kunlun 3.0 model, which is expected to debut in the upcoming Xiangjie S9, boasting integrated end-to-end capabilities synergized with LiDAR to significantly enhance autonomous driving functionality. In June, Wei Jianjun, Chairman of Great Wall Motors, showcased their latest intelligent driving system through a live demonstration in Chongqing, emphasizing a modular end-to-end architecture. As per reports in July, BYD's luxury brand Tengshi announced that they had successfully developed a "no map" end-to-end solution, marking the first phase towards achieving intelligent driving.
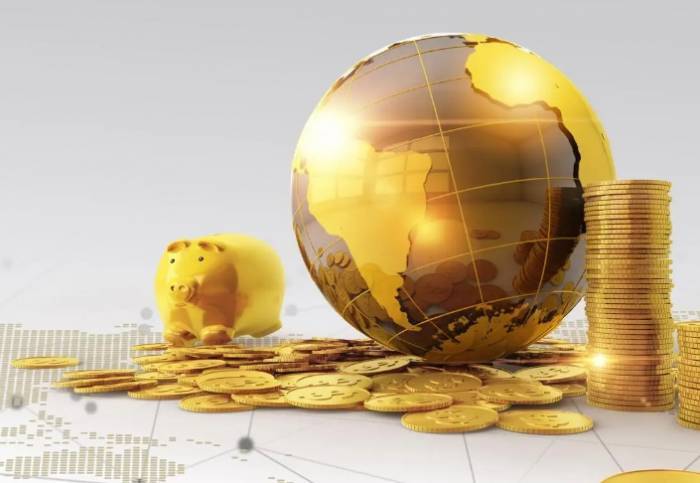
From these various product launches, it appears that many companies had been working on their end-to-end models since late last year, yet merely completing the prerequisites doesn’t guarantee immediate success. Following Tesla's lead, domestic firms are racing to catch up.
Xpeng and Huawei have adopted a segmented approach to their end-to-end technologies. They aim to replace sensing and planning modules independently with models while still employing engineer-defined rules to connect them. In contrast, Li Auto has made bolder strides by seeking to replace both the perception and planning modules with a sole model—an endeavor described as “taking a step back compared to Tesla yet moving a step further than local rivals.”
However, compared to Tesla's vision of an “inputting images, outputting controls” scheme, domestic methods for end-to-end implementation still rely on rules defined by engineers on the execution end. As highlighted by Jiang Haipeng, Senior Director of the Intelligent Platform Development at Great Wall Motors, the majority of algorithm companies or OEMs are exploring end-to-end methodologies, yet only three have genuinely committed to this framework.
Challenges remain, as pointed out by Lee Xiang, Chairman of Li Auto. He noted the need for skilled professionals specializing in end-to-end data training, high-quality datasets, and substantial computational power—key elements that continue to obstruct the industry’s progression in this area.
Algorithmic complexity, data quality, and computational resources present formidable hurdles regarding the practical implementation of end-to-end technologies. This dialogue surrounding whether end-to-end methodologies represent the future of intelligent driving remains inconclusive in the industry.
Yet, adversity often breeds innovation. Concerning computational requirements, Li Auto reported possessing 5,000 units of A100 and A800 training cards—twice the number Xpeng boasts—and profitability that supports rental services. As for data, Xpeng stated that its end-to-end model has access to training videos based on over one billion miles of cumulative mileage, logarithmically increasing its efficiency with a reported two-day iteration cycle.
For automakers striving for competitive advantage, realizing end-to-end models en masse is crucial—especially in securing a foothold within rapidly expanding urban autonomous navigation settings.
The vast potential of large models in vehicles extends beyond mere driving functions; tech firms are seizing the initiative as well. For instance, SenseTime has developed a general-purpose autonomous driving model called UniAD, which aims to unify perception and decision-making. This comprehensive visual solution aspires to mirror Tesla’s FSD initiative.
Currently, SenseTime's production-ready intelligent driving products can be seen across a variety of brands, including GAC Aion’s LX Plus and Hongqi. Features such as high-speed NoA have already started to gain traction.
Yuan Rong Qi Hang’s advanced driving platform, DeepRoute IO, has also been utilizing end-to-end models and is reportedly responsible for BYD's project on intelligent driving. Should this project yield success, it could potentially lead to a collaborative relationship that places Yuan Rong Qi Hang in the driver’s seat of China’s electric vehicle market.
However, while new entrants like NIO, Xpeng, and Li Auto concentrate their firepower on elevating intelligent driving capabilities, tech giants are exploring other applications for AI large models in automotive settings. This exploration spans not just autonomous driving; they are also aiming to improve intelligent cockpit experiences and streamline manufacturing, marketing, and customer engagement processes.
Large models within intelligent cockpits promise to revolutionize human-machine interactions by incorporating varied modalities such as voice, vision, and gestures, satisfactorily catering to user habits across different scenarios. NIO's large model NOMI GPT has recently launched, allowing for open-ended Q&A interactions, while tapping into third-party APIs facilitates a seamless user experience throughout NIO's ecosystem of products, services, and community engagements.
Similarly, iFlytek has rolled out a new intelligent cockpit system that integrates interactive functionalities for driving, communication, and entertainment. This advanced system has already found applications across more than ten automotive brands, including Chery and GWM.
Nevertheless, while the AI automotive sector is surging ahead with remarkable advancements, substantial limitations continue to hinder progress. According to a research report by the China Electric Vehicle Hundred Association titled "Artificial Intelligence Empowering New Energy Intelligent Vehicle Development," the lack of robust smart computing infrastructure is a primary constraint on accelerating the development of intelligent, connected vehicles. As vehicles and AI become deeply intertwined, the demand for advanced computational capabilities is skyrocketing, particularly for end-to-end driving and intelligent cabins.
To illustrate, the required computing power for the intelligent driving end-to-end technology pathway is pegged at 1 EFLOPS, advocating for Li Auto to scale to over 100 EFLOPS. The computational needs for large model training for intelligent cabins require upwards of 10 EFLOPS, with specialized model training and fine-tuning ranging from hundreds to thousands of PFLOPS.
As for computational provision, China's automotive companies average around 3 EFLOPS—markedly lower when contrasted with Tesla's 100 EFLOPS. Even major telecommunications firms collectively forecast only 53 EFLOPS of computational capacity available by the end of 2024. A lack of computational resources stunting the rapid iteration of intelligent driving algorithms in the nation remains a persistent issue.
The term “mature” computational power refers to systems like NVIDIA’s, encompassing both chips and software ecosystems. However, due to U.S. export restrictions, China's AI initiatives heavily rely on existing chips rather than innovating new ones, limiting the growth of this “mature” computational capacity.
On the flip side, “immature” computational power comprises offerings from companies like Huawei and Cambricon, which possess chip technology but lack a comprehensive software ecosystem. These limitations render software development cumbersome, leading to slow expansions of computational infrastructures and inefficient application usage. For these reasons, elevating “immature” computational power through better software ecosystems and addressing hardware constraints remains crucial for the industry’s advancement.
Leave a Reply